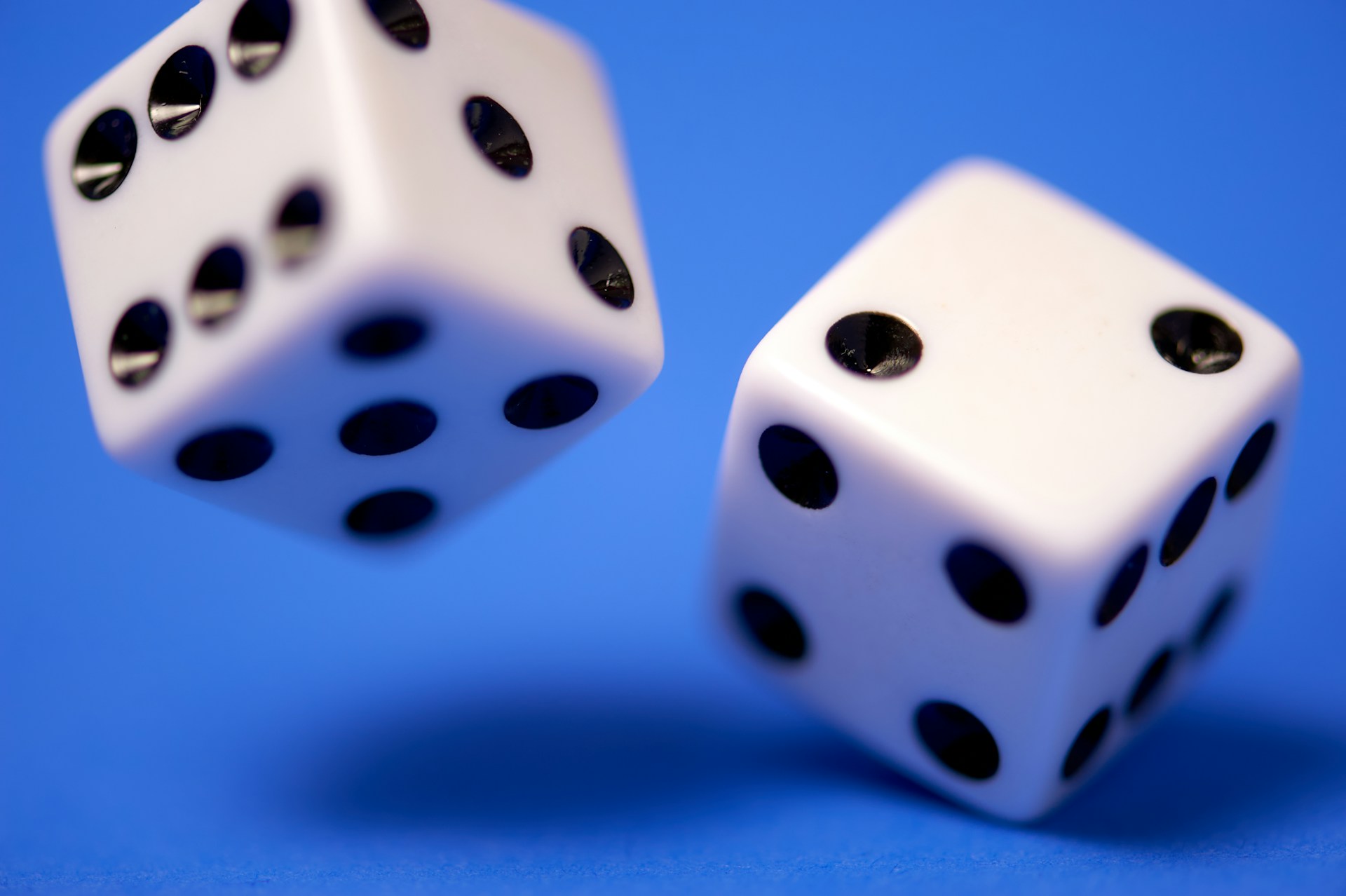
Chaos to Clarity: Probabilistic Linking in OSINT
An overview of probabilist linking, a statistical approach for bringing order and turning thousands of disparate, fragmented records into actionable insights.
Author: Sham Ahmed (Public Insights)
Open source intelligence (OSINT) relies on piecing together information from disparate sources to create a coherent picture of individuals, businesses, or activities. However, linking records across datasets that lack unique identifiers presents significant challenges. Many individuals share commonalities such as name and age, so finding the right social profile or public record connected to the correct entity can require extensive research. Probabilistic record linkage tools offer an opportunity to streamline this process and cut out the noise, providing scalable, accurate, and explainable solutions.
What is Probabilistic Record Linkage?
Probabilistic record linkage is a statistical approach that estimates the likelihood that records from different datasets refer to the same entity. Unlike deterministic matching, which requires exact matches on fields like name or date of birth, probabilistic methods accommodate variations, misspellings, and incomplete data. By leveraging statistical models, it quantifies the match probability, empowering investigators to work with more nuanced and ambiguous datasets.
Why Probabilistic Record Linkage Tools Matter
Tools implementing probabilistic record linkage offer a range of benefits for OSINT practitioners:
- Scalability: Capable of linking millions of records quickly, these tools can handle vast datasets often encountered in OSINT investigations, first identifying close matches and then predicting the likelihood that they relate to the right entity.
- Customisability: Many tools allow for defining fuzzy matching logic and term frequency adjustments to enhance accuracy for specific datasets. This can be adjusted depending on the uniqueness of a name or the quantity of results initially returned.
- Explainability: Advanced tools generate intuitive explanations of match probabilities and model parameters in simple terms, fostering transparency. This also ensures a consistent approach that removes human bias.
- Flexibility: They support deduplication, multi-dataset linkage, and user-defined comparison functions, adapting to various investigative needs.
Applications in OSINT Investigations
Probabilistic record linkage tools can significantly enhance OSINT workflows by:
- Connecting Public Records: Some automated OSINT tools aggregate records from diverse sources where data has different fields and quality standards. For example, Cradle looks at planning records, which in some council areas include an initialised first name and full surname. Matching this to the electoral roll, which has a full name and date of birth, isn’t always straightforward, particularly if the planning record is for a property that isn’t the address they are registered to vote at, such as a holiday home or rental. Probabilistic linkage ensures these records are connected to the correct individual, even when names are misspelt or addresses are outdated.
- Social Media Analysis: Linking social media profiles across platforms is a key OSINT task. Some users will use nicknames for one account, such as Instagram, while using their full name for professional purposes on LinkedIn. Social media platforms also sometimes use different ways to specify locations, such as LinkedIn using broader areas. Linkage tools can correlate these differences by comparing fields such as names, usernames, locations, and email addresses. They provide match probabilities, enabling analysts to focus on the most likely connections.
- Uncovering Networks: By linking individuals to addresses, businesses, and affiliations, these tools aid in uncovering networks of relationships that might otherwise remain hidden. Discovering a second address based on a high match can lead to the discovery of other individuals linked to that property, which can support the investigation of organised crime, fraud, and corporate misconduct.
A Case Study: Linking Public Records
Imagine an investigator tasked with uncovering the assets of a subject suspected of fraud. Publicly available data might include:
- Electoral Roll: Lists the subject’s residential address.
- Planning Permissions: Highlights property ownership or renovations.
- Insolvency Records: Identifies financial history.
Using a probabilistic linkage tool, the investigator can connect these records to create a unified profile, even if there are variations in the subject’s name or discrepancies in the address format. The resulting dataset offers a comprehensive view of the subject’s assets and financial activities.
Empowering Analysts, Not Replacing Them
While these tools automate much of the record linkage process, it’s important to note that decision-making remains firmly in the hands of analysts. Probabilistic matching provides estimates, not definitive conclusions. Analysts review these results, applying their expertise to determine the validity of the connections. The tool provides a list of assessed results, streamlining the decision-making process, but shouldn’t be trusted to get it right every time. This partnership between human intelligence and machine efficiency ensures investigations are both thorough and accurate.
Spotlight on Splink
One notable tool for probabilistic record linkage space is Splink, developed by the UK Ministry of Justice (MoJ). Splink was created as part of the MoJ’s Data First initiative, a programme aimed at improving the quality of administrative data for research and analysis across government departments. Splink was specifically built to address challenges associated with linking tens of millions of records concerning UK citizens lacking unique identifiers, such as a national ID card number.
Splink’s capabilities include:
- Scalability: Able to link millions of records in minutes, Splink is optimised for large-scale data linkage tasks.
- Explainability: By implementing Fellegi-Sunter’s statistical model, it generates intuitive reports and match probabilities that are easy to interpret.
- Customisability: Users can define comparison functions, apply term frequency adjustments, and tailor fuzzy matching logic to suit specific datasets.
- Free Access: Splink’s open-source nature as a command-line tool has enabled effective collaboration across government departments and academia.
The MoJ successfully used Splink to tackle linkage problems involving up to 15 million records with a runtime of under an hour. This same process can be used for tools that scan large amounts of fragmented publicly available information.
Conclusion
Probabilistic record linkage has significant potential for OSINT practitioners seeking to assess data at scale. Tools implementing this approach like Splink exemplify how this approach can bridge gaps in fragmented datasets to make the intelligence picture clearer, faster. By combining scalability, accuracy, and transparency, these tools empower OSINT practitioners to uncover connections that drive actionable intelligence.